Seminar in Psychometrics
Robust Bayesian meta-analysis: A framework for addressing publication bias with model-averaging
Date and time: March 30, 2021
Abstract. Publication bias poses a significant threat to meta-analyses - the gold standard of evidence. To alleviate the problem, a variety of publication bias adjustment methods was suggested. However, it is nearly impossible to select the correct method when the data generating process is unknown, which is usually the case, since no existing method performs well in a wide range of conditions. To address this issue, we developed a Robust Bayesian meta-analysis (RoBMA) framework. RoBMA allows us to combine different publication bias adjustment models in a coherent Bayesian way. Apart from obtaining the model-averaged estimates, RoBMA provides Bayes factor tests for presence or absence of the meta-analytic effect, heterogeneity, and publication bias. In this talk, I provide a conceptual introduction to Bayesian model-averaging in the context of meta-analyses, illustrate the RoBMA framework on an applied example, and demonstrate the performance of the method on real and simulated datasets.
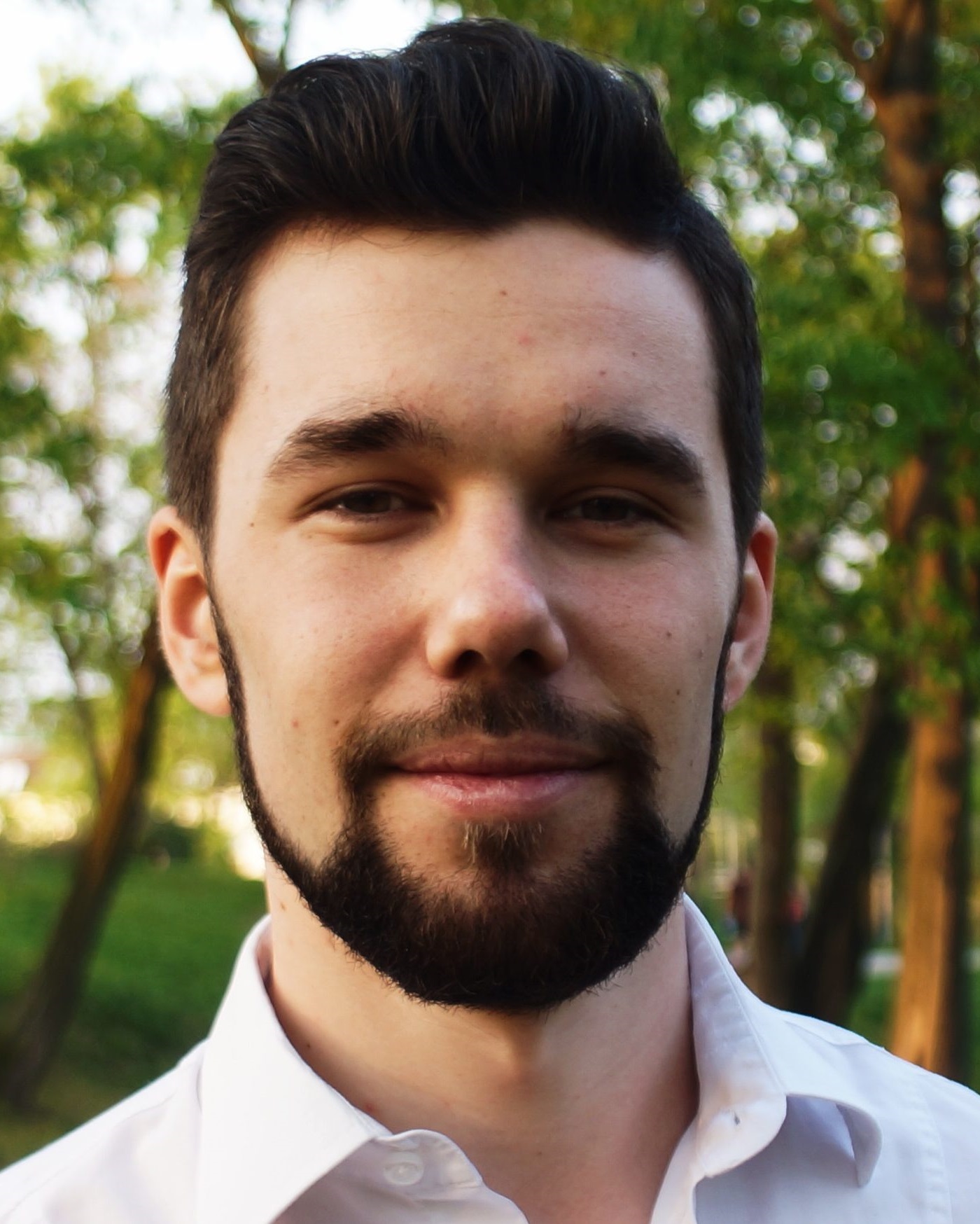